/dev/null
Where things I felt, heard, read or seen can, should or must be dropped. Blog name origin.
Thursday 4 July 2019
Monday 16 July 2018
Chromium OS for Raspberry Pi SBCs Is Making a Comeback Soon, Better Than Ever
Chromium OS for Raspberry Pi SBCs Is Making a Comeback Soon, Better Than Ever
Jul 13, 2018, 14:00 (0 Talkback[s]) (Other stories by Marius Nestor)Now that Flint OS is "gone," and there's still no feasible way of getting pure Chromium OS running on your tiny single-board computer, Keith Callahan announced today that he is reviving the Chromium OS for SBCs project in an attempt to offer the community an easy way of installing the open-source Chromium OS operating system on Raspberry Pi SBCs. Callahan told Softpedia that he is currently working hard on the new Chromium OS for SBCs 1.0 (Codename Weinreich) release, which should hit the streets near the Christmas holidays, with initial support for Raspberry Pi 2, Raspberry Pi 3 Model B, and the new Raspberry Pi 3 Model B+ boards.
Read the full article here by Linux Today
Guido van Rossum retires as Python's BDFL 😱
[python-committers] Transfer of power
Guido van Rossum guido at python.orgThu Jul 12 10:57:35 EDT 2018
Now that PEP 572 is done, I don't ever want to have to fight so hard for a PEP and find that so many people despise my decisions. I would like to remove myself entirely from the decision process. I'll still be there for a while as an ordinary core dev, and I'll still be available to mentor people -- possibly more available. But I'm basically giving myself a permanent vacation from being BDFL, and you all will be on your own. After all that's eventually going to happen regardless -- there's still that bus lurking around the corner, and I'm not getting younger... (I'll spare you the list of medical issues.) I am not going to appoint a successor. So what are you all going to do? Create a democracy? Anarchy? A dictatorship? A federation? I'm not worried about the day to day decisions in the issue tracker or on GitHub. Very rarely I get asked for an opinion, and usually it's not actually important. So this can just be dealt with as it has always been. The decisions that most matter are probably - How are PEPs decided - How are new core devs inducted We may be able to write up processes for these things as PEPs (maybe those PEPs will form a kind of constitution). But here's the catch. I'm going to try and let you all (the current committers) figure it out for yourselves. Note that there's still the CoC -- if you don't like that document your only option might be to leave this group voluntarily. Perhaps there are issues to decide like when should someone be kicked out (this could be banning people from python-dev or python-ideas too, since those are also covered by the CoC). Finally. A reminder that the archives of this list are public ( https://mail.python.org/pipermail/python-committers/) although membership is closed (limited to core devs). I'll still be here, but I'm trying to let you all figure something out for yourselves. I'm tired, and need a very long break. -- --Guido van Rossum (python.org/~guido) -------------- next part -------------- An HTML attachment was scrubbed... URL: <http://mail.python.org/pipermail/python-committers/attachments/20180712/30a41d64/attachment-0001.html>
More information about the python-committers mailing list
Read the full article here by Changelog
Live Twitch • Gros défis sur The Crew 2

Read the full article here by Le comptoir du hardware
Saturday 14 July 2018
Friday 13 July 2018
Wednesday 11 July 2018
Monday 2 July 2018
Install And Play Overwatch With Lutris On Ubuntu 18.04
Linuxconfig: Overwatch is one of the most popular PC games right now. It has a thriving esports scene and a community of millions playing it regularly worldwide.
Read the full article here by Linux Today
Friday 29 June 2018
MindForger - More Than A Notebook and Markdown IDE
MindForger - More Than A Notebook and Markdown IDE
Jun 29, 2018, 06:00 (0 Talkback[s]) (Other stories by FossMint)MindForger is a modern, free, open-source, privacy-focused and performance-driven Markdown IDE for creating, editing, and managing all types of notes.
Read the full article here by Linux Today
Thursday 28 June 2018
Google Doubles Down on Linux and Open Source
Read more of this story at Slashdot.
Read the full article here by Slashdot
Wednesday 27 June 2018
Google Opens Its Human-Sounding Duplex AI To Public Testing
Read more of this story at Slashdot.
Read the full article here by Slashdot
Saturday 23 June 2018
Leclerc: “L’ottavo tempo è magico, non ci posso credere”
Read the full article here by FormulaPassion.it
Wednesday 20 June 2018
AMD trolle joliement Intel et son 8086K
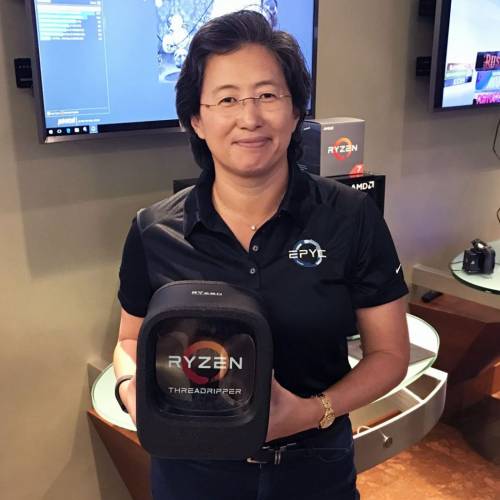
Test • Intel Z370 / Core i7-8700K / i5-8400 / i3-8350K
Coffee Lake, l'anti Ryzen d'Intel ? Durant les années 2000 et consécutivement à l'échec de Netburst, Intel a mis en place la stratégie du Tick Tock, co...Read the full article here by Le comptoir du hardware
Microsoft ports Windows 10, Linux to homegrown CPU design

Microsoft has ported Windows 10 and Linux to E2, its homegrown processor architecture it has spent years working on mostly in secret.
As well as the two operating systems, the US giant's researchers say they have also ported Busybox and FreeRTOS, plus a collection of toolkits for developing and building applications for the processor: the standard C/C++ and .NET Core runtime libraries, the Windows kernel debugger, Visual C++ 2017's command line tools, and .NET's just-in-time compiler RyuJIT.
Microsoft has also ported the widely used LLVM C/C++ compiler and debugger, and related C/C++ runtime libraries. The team wanted to demonstrate that programmers do not need to rewrite their software for the experimental chipset, and that instead programs just need to be recompiled - then they are ready to roll on the new technology.
I had no idea Microsoft was working on its own instruction set - even if only for research purposes. The Register has some more information on what E2 is like.
The Register understands from people familiar with its development that prototype E2 processors exist in the form of FPGAs - chips with reprogrammable circuitry that are typically used during the development of chips. For example, a dual-core implementation on Xilinx FPGAs exists, clocked at 50MHz. The team has also developed a cycle-accurate simulator capable of booting Windows and Linux, and running applications.
Qualcomm researchers were evaluating two EDGE chip designs with Microsoft: a small R0 core, and an R1 core running up to 2GHz fabricated using a 10nm process. The project, we must stress, is very much a work in progress.
It seems to be a radical departure from the norm, and I'm very interested to see where this will lead.
Read the full article here by OSNews
Tuesday 19 June 2018
Wait Management Center
Wait Management Center
Tuesday, June 19th, 2018 :: 12:01 am
Read the full article here by Buttersafe
Monday 18 June 2018
EXT4 fscrypt vs. eCryptfs vs. LUKS dm-crypt Benchmarks
Given the recent advancements of the EXT4 file-system with its native file-system encryption support provided by the fscrypt framework, here are benchmarks comparing the performance of an EXT4 file-system with no encryption, fscrypt-based encryption, eCryptfs-based encryption, and a LUKS dm-crypt encrypted volume.
For those wondering how these different file-system encryption options compare for performance, I ran some fresh benchmarks using a Linux 4.18 development kernel as of 14 June from the Linux Git tree. The options tested were EXT4, fscrypt, eCryptfs, and LUKS dm-crypt encryption with the EXT4 file-system and tested with the defaults unless otherwise noted. A Toshiba TR150 SATA 3.0 SSD was used as the drive under test for all of the benchmarking. Ubuntu 18.04 LTS was the basis for this benchmarking aside from the Linux kernel upgrade.
All of thes Linux file-system encryption benchmarks were carried out in a standardized and fully reproducible manner using the open-source Phoronix Test Suite benchmarking software.
Read the full article here by Phoronix
Tuesday 12 June 2018
F2FS File-System Gets Discard Improvements, Nobarrier Fsync Mode For Linux 4.18
Flash-Friendly File-System (F2FS) maintainer Jaegeuk Kim has submitted the file-system updates intended for the Linux 4.18 kernel.
This flash storage optimized file-system has improvements around its discard/fstrim support, including the splitting of large discard commands for better responsiveness. There is also a new
fsync_mode=nobarriermount option available for reducing the number of cache flushes.
F2FS for Linux 4.18 also has improved sanity checks, various code clean-ups, and at least six known bug fixes.
The complete list of F2FS file-system changes for Linux 4.18 can be found via
the kernel mailing list.
Read the full article here by Phoronix
Monday 11 June 2018
How to Android without Google

This guide shows how to install LineageOS without GApps with the help of signature spoofing and microG, so that you can have Push Notifications, Location Services and the like, without needing to have Google Play Services installed (without Google-anything for that matter).
It was made possible by the hard work of creators, maintainers and community around LineageOS, microG, XPosedFramework, F-Droid, Yalp Store and many others.
Exactly what it says on the tin.
Read the full article here by OSNews